Artificial Intelligence revolutionizes how we study proteins
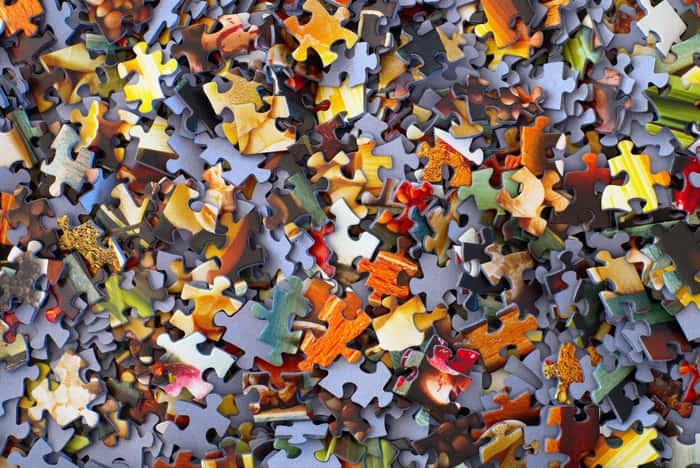
Artificial Intelligence revolutionizes how we study proteins
Predicting the shape of a protein from its amino acid sequence is one of biology’s greatest puzzles, known as the protein folding problem. The three dimensional, or globular shape of a protein is essential to its cellular function, which means that visualizing protein structures is vital to numerous fields across the biological sciences. However, there are significant challenges to solving protein conformations through lab-based methods. Huge diversity of amino acid combinations, health risks from X-Ray crystallography, and rapid degradation of protein samples has led to slow and often frustrating progress for biochemists over the last fifty years (1). The next generation of methods in protein structure elucidation hinge on advanced computing power and artificial intelligence.
The Frontrunner: AlphaFold by DeepMind
Early this year, a team from DeepMind published their newest AI protein structure prediction system, AlphaFold. The system was developed as an entry by DeepMind to the Critical Assessment of protein Structure Prediction (CASP) competition and won the first place prize at CASP13 held in 2018 (2) and again at CASP14 in 2020. AlphaFold uses three deep-learning mechanisms to 1) predict the distance between amino acid pairs, 2) estimate the accuracy of a potential structure, and 3) generate 3-D protein structures (3). Model predictions from AlphaFold improved on previous methods, making significant progress in determining protein configurations by artificial intelligence (2). AlphaFold neural networks are trained to predict the distance and the bond angle between amino acids for an unknown structure. All the measurement predictions are then combined to give the proposed final structure an overall accuracy score.
Future innovation builds on traditional methods
The incremental, but foundational, work by biochemists in the 20th and 21st centuries generated databases full of known protein sequences and structures. In order to predict an unknown structure, AlphaFold and other deep-learning methods require a vast pool of known data from databases such as the Protein Data Bank. Conformations with the most accurate scores from AlphaFold algorithms are compared against published protein structures (3). AlphaFold’s success, coupled with increasing availability of new data, has expanded the horizon of possibility in protein engineering, from groundbreaking new drugs to imaginative biochemical weaponry.
In the next installment of our three-part series, we’ll explore some of these potential applications for AlphaFold and discuss their impacts on medicine, the economy, and human rights.
References
- Jonathan C. Brooks-Bartlett & Elspeth F. Garman (2015) The Nobel Science: One Hundred Years of Crystallography, Interdisciplinary Science Reviews, 40:3, 244-264, https://doi.org/10.1179/0308018815Z.000000000116
- Senior, A., Jumper, J., Hassabis, D., & Kohli, P. (2020). AlphaFold: Using AI for scientific discovery. Retrieved from https://deepmind.com/blog/article/AlphaFold-Using-AI-for-scientific-discovery
- Senior, AW, Evans, R, Jumper, J, et al. Protein structure prediction using multiple deep neural networks in the 13th Critical Assessment of Protein Structure Prediction (CASP13). Proteins. 2019; 87: 1141– 1148. https://doi.org/10.1002/prot.25834
- Most Viewed Blog Articles (5)
- Company News (285)
- Emerging Technologies (64)
- Microbiology and Life Science News (93)
- Water and Fluid Separation News (97)
- Filtration Resources (93)
- Product News (19)